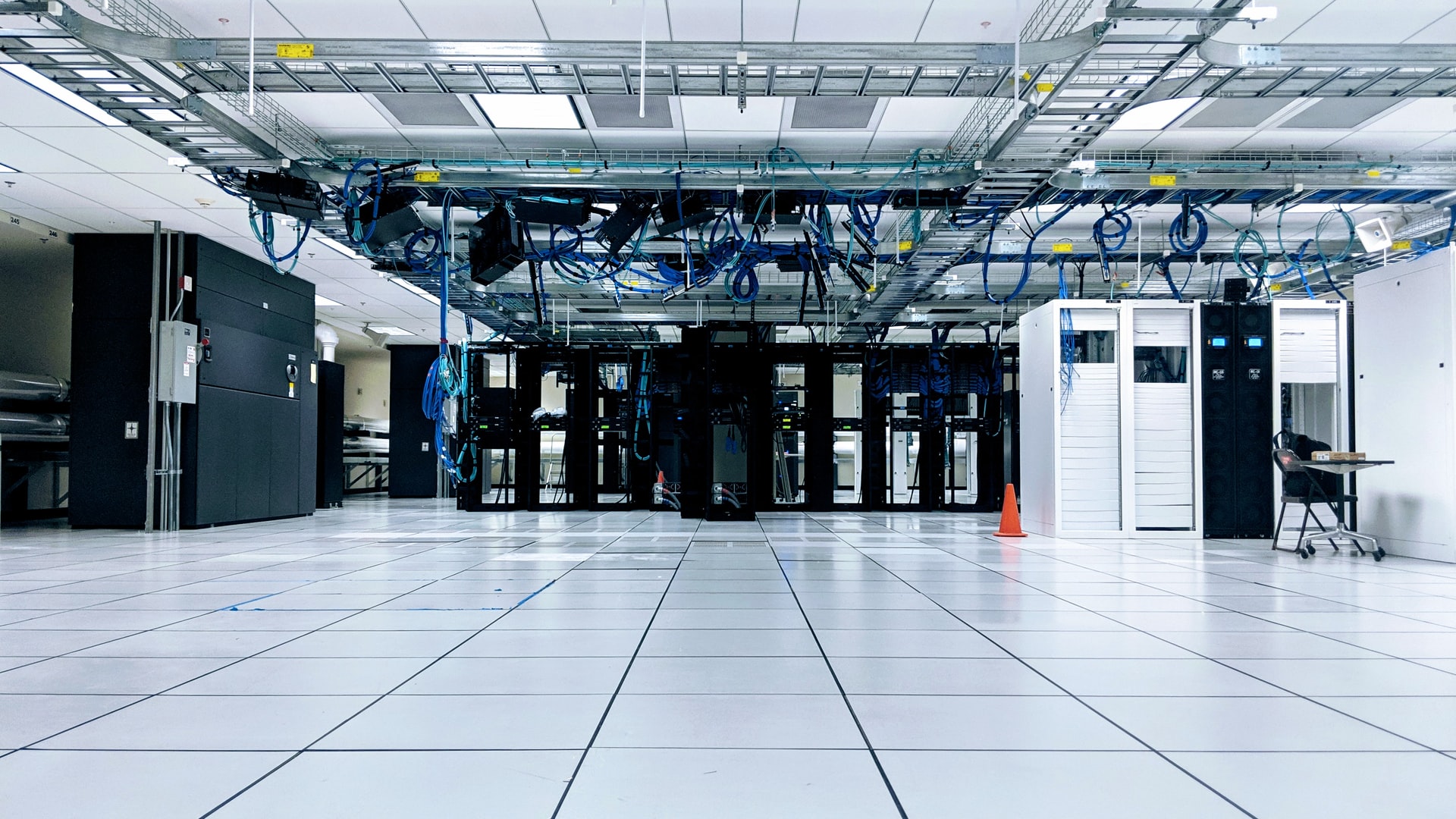
These Limitations of AI May Be Holding Businesses Back
March 3, 2022 - Revolutionized Team
Revolutionized is reader-supported. When you buy through links on our site, we may earn an affiliate commission. Learn more here.
Artificial intelligence has the potential to revolutionize how the business world analyzes data. With the right AI model, it’s possible to make better predictions and uncover patterns that conventional analytic strategies may miss. However, as the popularity of AI has grown, businesses have also had to confront some of the significant limitations of AI.
The Potential and Growing Adoption of AI
Artificial intelligence has the potential to add significant value across a wide variety of sectors. Businesses in retail, oil and gas, and logistics can all benefit from the implementation of AI algorithms. Often, these algorithms take advantage of data that these sectors already have access to.
New use-cases for AI are being pioneered all the time. Even if a business can’t find a reason to adopt AI today, it may find one next year.
AI has the potential to generate trillions of dollars of value over the next few decades. Adoption of the tech could potentially revolutionize how businesses process data and plan automation.
Big Tech companies, like Facebook, Amazon and Google, are leading the way on AI adoption. Even small businesses are beginning to invest in ready-to-use AI tools as the value of the technology becomes more apparent.
As a result, it’s more important than ever that businesses and researchers grapple with the major limitations of AI.
1. The Data Problem
An effective AI algorithm requires a vast amount of information. Otherwise, the algorithm may not function correctly — or its analysis may not be accurate.
For example, the Inception V3 model from Google, used to classify images, has around 24 million parameters and requires 1.2 million data points for training. In the case of this model, that means researchers need to collect and label 1.2 million images.
If any of these images are low quality or incorrectly labeled, the model may not produce a useful algorithm. Cleaning and labeling data is necessary, but can further increase needed labor.
Not every model will require this much data, but data-gathering can be a bottleneck even when just a few hundred or thousand data points are needed.
For example, a new business may be using AI to create a new sales forecasting algorithm. If the company has been in business for just 2 years, it may only have so much data on sales and marketing information.
The AI’s forecasting ability may be limited. Noise in the data set may be overrepresented, leading the model to make predictions that are inaccurate, confusing or not useful.
The High Cost of Prepping Data for Use by AI
Gathering, organizing and labeling data can also be labor-intensive, no matter what you’re trying to accomplish. AI researchers often rely on existing datasets to reduce the amount of work needed to experiment. Businesses may not have this luxury.
While AI often excels at analyzing unstructured data — information that’s disorganized, poorly labeled or not structured in a way that makes analysis easy — this data often can’t be used in training. As a result, the cost-saving potential of AI may only be realized only after an expensive training and development period.
The data problem is less of a problem for businesses that do not develop their own AI algorithms and instead rely on other companies in their niche that have designed ready-to-use algorithms or tools. However, in-house AI development could be out of reach for many small businesses due to the amount and quality of data they would need.
2. The Bias Problem
A sufficiently trained machine learning model can only replicate reality. For some applications, this isn’t necessarily a problem — but in many cases, the bias that exists in a data set will exist in the model, as well.
There are many examples of how this has already happened to businesses in a variety of industries.
For example, US court systems use an AI algorithm called COMPAS (Correctional Offender Management Profiling for Alternative Sanctions) to predict the likelihood that a defendant would become a recidivist.
The model was trained on data with patterns of discrimination and no controls for these patterns. As a result, it was twice as likely to predict recidivism for black defendants as it was to predict recidivism for white defendants.
The world of Big Tech is also vulnerable to machine bias. Amazon once used AI as the basis for a resume-scanning algorithm. It abandoned this algorithm after it became apparent the model was assigning lower scores to candidates who had attended women’s colleges.
In healthcare, diagnosis algorithms may underdiagnose patients based on factors like sex, race or income — even if the algorithm doesn’t have information to patient demographic data.
If bias exists in the real-world data set that you use to train a model, it is likely that bias will exist in the model as well.
AI Algorithms May Help “Launder” Bias
End-users tend to think of computers as being unbiased. As a result, it’s possible that the model may help smuggle bias past individuals who are trained to identify bias. As a result, a biased algorithm can cause even more harm than business as usual, if workers are willing to accept a biased result from an apparently neutral machine.
This bias isn’t inevitable. Computer scientists are constantly working on techniques and tools that will allow businesses to reduce machine bias. With careful training oversight, it’s possible to identify and manage or eliminate bais in AI algorithms.
However, this oversight costs time, money and labor. It also requires an AI analyst with the skills necessary to correct for bias. As a result, many businesses may choose to delay AI development or simply tolerate the risk of creating a biased algorithm.
As AI becomes more widely adopted and more necessary for businesses to stay competitive, machine bias may become a much bigger problem.
3. The Time Problem
Training a new AI model takes a great amount of time and processing power. Even as computers become much more powerful, AI models are still likely to be a major time commitment.
AI models require high-power hardware that can’t be used for anything else while the model is being trained. As a result, they’re often expensive to create.
A shortage of high-powered computer hardware isn’t helping the problem. In addition to being used for AI training, this hardware is also used for crypto mining. The growing value of cryptocurrency has driven up hardware prices and created a new shortage as crypto miners invest more in their mining set-ups.
As a result, the hardware necessary for AI training has become a significant investment, one out of the reach of many small businesses.
4. The Talent Problem
In addition to a significant labor shortage, many businesses also face a serious talent gap. Fields related to computer science, in particular, are struggling with a shortage of skilled workers. In general, if you need a cybersecurity expert, IT officer or AI data scientist, you may find there are very few qualified applicants for a new position.
These talent shortages have gotten significantly worse over the past few years. There’s also no evidence that the labor gap is going to start shrinking anytime soon. Workers in most in-demand fields are also struggling with a burnout crisis. As businesses expect more from the skilled workers that they do have access to, these crises may get worse.
There are strategies that can reduce the talent gap, but many of them only work in the long term. For example, many businesses and organizations are beginning to invest in AI training and education programs. These will help build a stronger pipeline for AI talent. Investments in education typically take years to pay off, however. Businesses may not see the talent gap shrink until long after they first began to invest in new AI experts.
5. The Security Problem
While AI has the potential to revolutionize cybersecurity through new security tools and technologies, a misapplied AI model could create new vulnerabilities.
AI systems can be vulnerable to intentional attacks. Hackers can generate audio that sounds like speech to an audio recognition algorithm but not humans, for example. This would allow hackers to potentially bypass voice-recognition security with the right audio. A similar attack can work against image-recognition and face-recognition algorithms.
Adversarial attacks like these enable hackers to leverage quirks in AI algorithms against the system. These attacks use data that looks innocuous to a human observer to fool an otherwise effective AI system.
Businesses Should Prepare to Manage the Limitations of AI
As AI becomes more commonplace, businesses need to be aware of the drawbacks that come with the technology. The high cost of AI, its data requirements and potential security vulnerabilities all make the effective use of AI more challenging.
All of these limitations of AI can be overcome with both individual action and structural changes. However, businesses will need to begin working on these strategies right now, before AI becomes a normal tool in the business world.
Revolutionized is reader-supported. When you buy through links on our site, we may earn an affiliate commission. Learn more here.