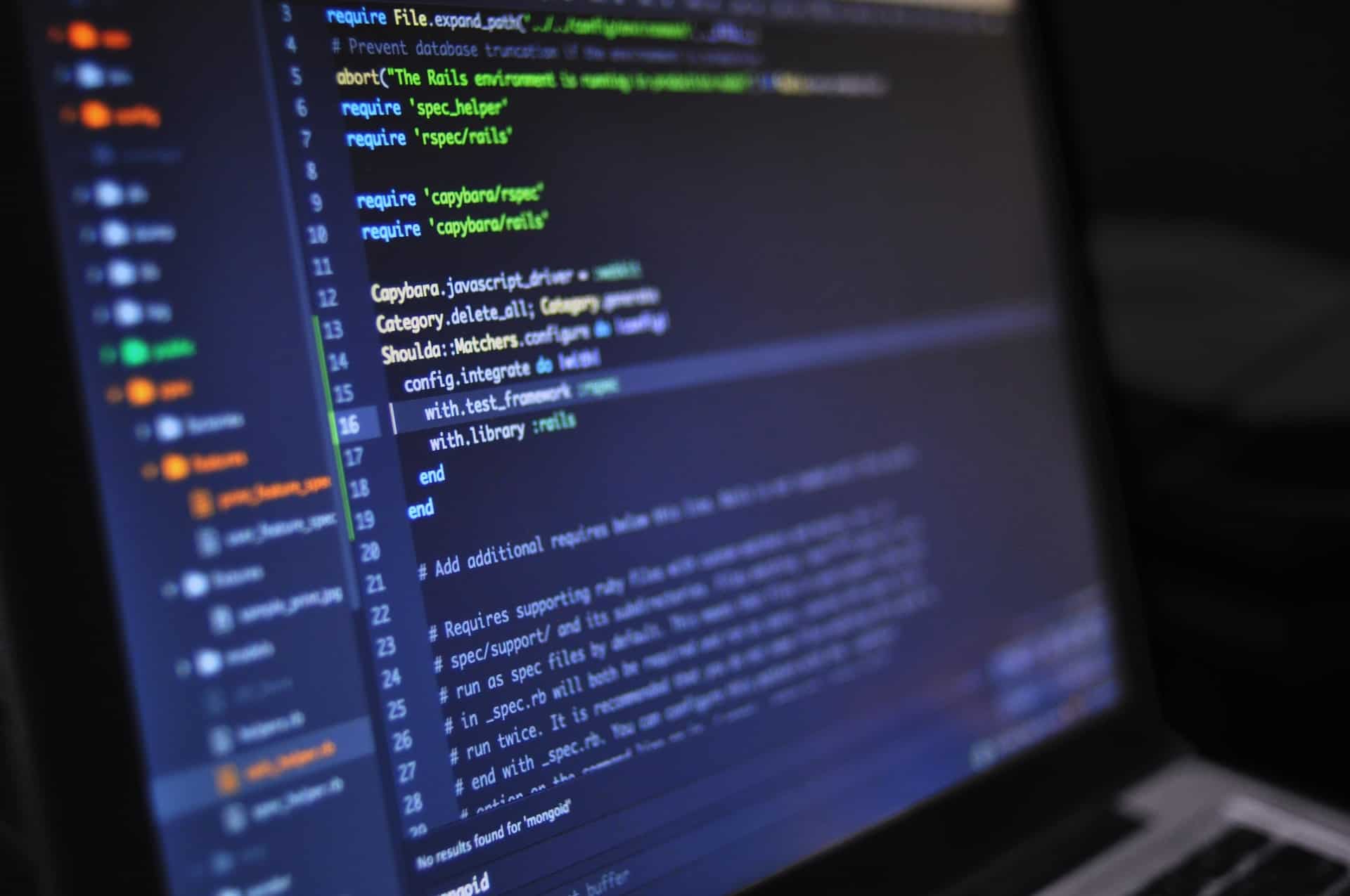
How Will Machine Learning Improve the Internet of Things?
March 4, 2021 - Emily Newton
Revolutionized is reader-supported. When you buy through links on our site, we may earn an affiliate commission. Learn more here.
The Internet of Things (IoT) encompasses a tremendous assortment of connected devices — from washing machines to stethoscopes and almost anything else that comes to mind. These gadgets have advanced technology working in the background, usually in the form of machine learning algorithms that bring context to data. Here are some exciting examples of how machine learning for IoT products could make them more valuable than ever.
Machine Learning Makes Smart Speakers More Capable
Amazon smart speakers helped make IoT technologies mainstream by bringing them into homes. These IoT gadgets get progressively more advanced, and that’s mainly due to machine learning.
For instance, tens of thousands of skills expand what the Alexa smart assistant can do. However, machine learning lets people access them without remembering the exact skill names. A machine learning model helps Alexa hear a person’s requests in context, then choose the best skill to help them.
A more recent example concerns using machine learning to help Alexa get smarter by filling gaps in what it already knows. It already engages in self-learning by picking up feedback clues from users. For example, a person might interrupt Alexa if the smart speaker does not respond as expected or say, “No, not that” or, “That’s wrong.”
This newer example of machine learning in an IoT device makes Alexa ask follow-up questions after a user gives a relevant prompt. The assistant then uses a person’s responses to behave more relevantly during future interactions.
Other smart speakers use machine learning too. However, Amazon is exceptionally transparent about using machine learning to improve its tech and what enhancements users should expect.
IoT Gadgets Get More Accurate, Faster, and Increasingly Secure With Machine Learning
Deep learning is a machine learning subset based on algorithms inspired by the human brain’s structure and functionality. That’s why people working with deep learning often discuss artificial neural networks.
MIT researchers recently devised a system to bring deep learning networks to new, smaller places. It’s called MCUNet and offers a way to design compact neural networks that make IoT devices work faster and with fewer errors, even when the gadgets have limited processing power. This combination of machine learning and IoT technology could bolster data security, too.
Machine learning instances involving neural networking usually process information in the cloud. That’s a necessity because IoT devices typically run with microcontrollers. They have less than one-thousandth the memory of a smartphone, making on-device processing of complex machine learning impossible.
However, MCUNet facilitated neural network deployment directly on the devices. Data stays safer because processing no longer happens in the cloud, which poses hacking vulnerabilities.
First, the researchers designed an interference engine to direct resource management, similar to an operating system. Then, they made a neural architecture search algorithm called TinyNAS. The interference engine runs a specific neural network structure that TinyNAS selects.
A crucial detail about TinyNAS is that it optimizes the search space to work well with various microcontroller sizes. Thus, the resulting neural networks provide the optimal performance based on the microcontroller used by a device.
It’s too early to say what this development means regarding machine learning for IoT. The team’s advancements are undoubtedly promising, but the potential will be more evident through real-world use cases. There’s continual interest in IoT security, though, so this research could give a glimpse into developing innovative gadgets that do not need cloud-based processing.
Machine Learning Facilitates Better Data Usage in Industrial Settings
Using the IoT for business reasons has become such a widespread phenomenon that people began referring to it with a subcategory: The Industrial Internet of Things (IIoT).
In today’s most advanced facilities, connected machines communicate with each other from across a factory floor. Smart robots work alongside humans or retrieve more supplies when they run out. Intelligent machines can tell managers crucial information about cycle times, daily output, error rates, and other concerns.
However, it’s not enough to merely collect the data. Machine learning can help users make sense of the statistics and recognize important patterns that guide decision-making. For example, statistics say the IoT will cause a 40% reduction in manufacturing equipment maintenance costs by 2025. That’s because more company leaders use predictive tools to help them identify problems before machines fail. Machine learning models can make people more aware of overall machine health.
Regression models can predict the equipment’s remaining useful lifetime, while classification models analyze the likelihood of specific failures within a given window. People develop regression models to determine continuous quality and build classification models to assign labels to data. Using the equipment example, maintained expected performance is the desired continuous quality, and labels determine whether data indicates normal operation or a fault.
Machine learning and IoT advancements could also pay off in areas that influence public health and a company’s reputation, such as food safety. Suppose company leaders collect data about aspects like temperature fluctuations, worker hygiene, and handling at all stages of the supply chain. It then becomes easier to catch issues of possible contamination before goods reach the marketplace and risk making people sick.
Machine Learning and IoT Facilitate Faster Medical Diagnoses and Fewer Complications
Many medical centers have broad assortments of IoT devices that improve patient care. For example, wearable gadgets make providers aware of problems before people show worrisome symptoms. Some patients use connected gadgets to see providers from their homes, removing the need to travel to a facility. When machine learning processes the available data, physicians can make faster, more personalized decisions to help patients.
One recent example showed how doctors could use smart medical gadgets trained with machine learning to improve diagnosis accuracy during the COVID-19 pandemic. Researchers trained three types of machine learning models to assist with COVID-19 diagnoses. The most successful of the three achieved an accuracy rate of up to 95%.
The researchers’ system involved collecting data from smart medical equipment, sending it to the cloud for processing, then transferring the information to the machine learning models. The people involved believe this setup could support making better patient care decisions while tackling problems that could exacerbate mortality rates, such as hospital overcrowding.
Other examples of machine learning for IoT applications reduce adverse outcomes. A wearable tool might analyze someone’s gait and balance abilities, then use the findings to calculate a fall-risk score. It could help a clinician decide if it’s safe to send a person home while they recover from a previous fall or similar incident.
Machine Learning for IoT Takes Connected Cities Into the Future
Today’s cities are progressively more advanced, and machine learning is one of the main drivers behind the advancements. Smart traffic lights respond to evolving movement patterns. Integrated building systems assess the best ways to save energy while simultaneously keeping the premises secured against unauthorized occupants.
Machine learning also enables connected devices to view environments in context, providing vital content to human decision-makers. Smart cameras can track occupancy levels, telling authorities when public events get too crowded. They can also pick up on details that may put others at risk. A newly launched camera equipped with machine learning and computer vision can tell how long people linger in an area or whether they’re wearing face masks.
The camera also detects car accidents, unknown individuals, and whether people show interest in displayed merchandise. People who decide to use products like these must take care to avoid ethical concerns. Which actions might cross the line between keeping city-dwellers safe and invading their privacy or profiling them in potentially prejudicial ways?
Advanced technologies for smart cities are not always camera-related, however. Some streetlights have sensors that turn them on or off as people move into or leave an area. If those products include machine learning algorithms, they could tell city authorities how often people populate certain public places and which times of day they visit most often. Information like this could come from how frequently the lights activate or stay off.
Machine Learning Accelerates IoT Potential
The Internet of Things alone has dramatically changed how people use and benefit from technology. However, room for improvement always exists. Machine learning is not a guaranteed way to enhance the IoT. However, these examples highlight some of the fascinating ongoing research and applications that let the two technologies create a smooth pairing.
Revolutionized is reader-supported. When you buy through links on our site, we may earn an affiliate commission. Learn more here.
Author
Emily Newton
Emily Newton is a technology and industrial journalist and the Editor in Chief of Revolutionized. She manages the sites publishing schedule, SEO optimization and content strategy. Emily enjoys writing and researching articles about how technology is changing every industry. When she isn't working, Emily enjoys playing video games or curling up with a good book.